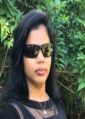
Meenalosini Vimal Cruz
Keene State College
Title: A robust automated brain tumor segmentation using NiftyNet
Biography
Biography: Meenalosini Vimal Cruz
Abstract
Glioblastoma Multiforme (GBM) is a fast growing aggressive cancer type which forms on the supportive tissue of the brain. The key treatment for this type of cancers is very important and early detection is desirable to extend the life expectancy of oncological patients. Magnetic Resonance Imaging (MRI) is a common and popular technique widely used to scan for these tumors and the MRI produces a large amount of 3D data which is usually a challenging problem. Moreover, the large spatial and structural variability in the tumors also increases the complexity of automatic segmentation. Automated segmentation is an essential condition in tumor segmentation for various quantitative analyses and still it remains to be a challenging problem due to the complex nature of MRI images. Hence in this research, a novel Convolutional Neural Network (CNN) framework called Niftynet is used to perform a robust segmentation. Niftynet, generally uses a smaller kernel of size 3x3 which is the base for a deeper architecture and involves the positive effects against overfitting problem. Further, this research contributes an additional pre-processing step which use intensity normalization for an effective tumor segmentation. The proposed framework is validated in the UCI primary tumor dataset and BIOGPS brain cancer dataset. The experimental results confirm that the proposed framework using Niftynet is more robust to segment the previously unseen tumors in MRI images, image-specific fine tuning and weight updation significantly improves the segmentation accuracy.